일 | 월 | 화 | 수 | 목 | 금 | 토 |
---|---|---|---|---|---|---|
1 | 2 | 3 | 4 | 5 | 6 | 7 |
8 | 9 | 10 | 11 | 12 | 13 | 14 |
15 | 16 | 17 | 18 | 19 | 20 | 21 |
22 | 23 | 24 | 25 | 26 | 27 | 28 |
29 | 30 |
Tags
- PySpark
- DataFrame Spark
- 언어모델
- spark #스파크
- 프로그래머스 파이썬
- tableau
- BigQuery
- pyspark오류
- SparkSQL
- spark explode
- 도커
- airflow
- spark df
- 태블로
- 코테
- 로컬 pyspark
- 데이터엔지니어링
- sparkdf
- 데이터 시각화
- Big Query
- 빅쿼리
- docker
- LLM
- Docker error
- ifkakao2020
- 도커오류
- dataframe
- 빅쿼리 튜닝
- 도커exec
- 시각화
Archives
- Today
- Total
SOGM'S Data
SPARK_02 : DATAFRAME_2 본문
SPARK DataFrame 조작 모음
from pyspark.sql import SparkSession
from pyspark.sql import Row
from pyspark.sql import functions as func
spark = SparkSession.builder.appName("FriendsByAge").getOrCreate()
lines = spark.read.option("header", "true").option("inferSchema", "true").csv("file:///SparkCourse/fakefriends-header.csv")
# Select only age and numFriends columns
friendsByAge = lines.select("age", "friends")
# From friendsByAge we group by "age" and then compute average
friendsByAge.groupBy("age").avg("friends").show()
# Sorted
friendsByAge.groupBy("age").avg("friends").sort("age").show()
# Formatted more nicely
friendsByAge.groupBy("age").agg(func.round(func.avg("friends"), 2)).sort("age").show()
# With a custom column name
friendsByAge.groupBy("age").agg(func.round(func.avg("friends"), 2)
.alias("friends_avg")).sort("age").show()
spark.stop()
특히 spark sql 의 function 모듈을 import 하여 agg를 통해 df를 조작하는 부분이 pandas의 apply와 비슷하다.
<예제: Word-counting , 단어 개수 세기>
영어로 된 텍스트 파일이 있다.
이 텍스트 파일에서 쓰인 단어의 수를 세보자.
1.필요 모듈 호출 및 SparkSession 초기화
from pyspark.sql import SparkSession
from pyspark.sql import functions as func
spark = SparkSession.builder.appName("WordCount").getOrCreate()
2. 데이터 조작 -> 스키마 없는 txtfile
inputDF = spark.read.text("file:///SparkCourse/book.txt")
words = inputDF.select(func.explode(func.split(inputDF.value, "\\W+")).alias("word"))
words.filter(words.word != "")
text파일을 불러온 뒤, select로 func 함수를 이용하여 expode한다.
여기서 중요한 점은, 스키마가 없는 최초의 dataframe을 가져올 때 row objects로 기본적으로 구성되어 있으며 단 하나의 'value'라는 이름의 칼럼을 가진다.
즉 inputDF.value 라는 칼럼을 스페이스나 문장부호 ( "\\W+")를 기준으로 split해주는 것이다.
이후 explode하는데 RDD의 FlatMap과 같은 기능이다. (각 요소들에 대해 여러 행을 생성)
*flatmap 과 map의 차이는 해당 블로그 참조 (https://eyeballs.tistory.com/148)
마지막으로 words DF의 word 칼럼이 공백인 경우 삭제한다.
3. 단어 개수세기
lowercaseWords = words.select(func.lower(words.word).alias("word"))
#소문자화
wordCounts = lowercaseWords.groupBy("word").count()
wordCountsSorted = wordCounts.sort("count")
wordCountsSorted.show(wordCountsSorted.count())
우선 lower()함수를 통해 소문자로 다 만들어준 뒤, groupby 집계값으로 단어별 빈도를 체크한다.
sort역시 count순으로 진행한 뒤 출력!
결과
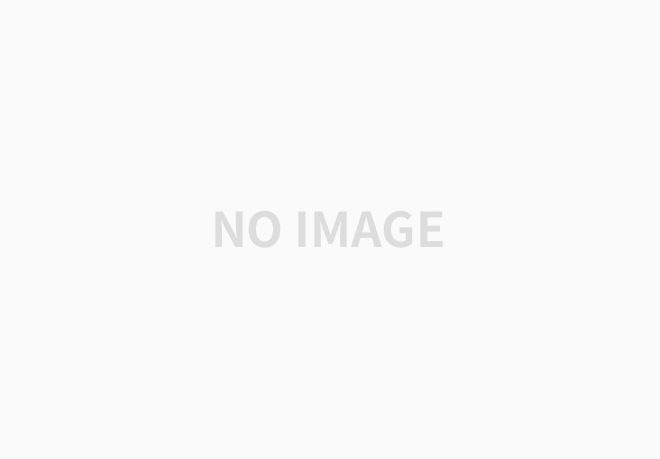
단어가 빈도 수 별로 잘 정렬되어짐을 확인할 수 있다.
'About Data > Engineering' 카테고리의 다른 글
SPARK BFS 구현 (0) | 2022.06.12 |
---|---|
SPARK_02 : DATAFRAME_1 (0) | 2022.02.17 |
SPARK_01 : RDD_2 (mapvalue, reduceByKey) (0) | 2022.02.06 |
SPARK_01 : RDD_1 (0) | 2022.01.11 |
로컬에 spark 설치후 pyspark 실행 오류 (3) | 2021.12.31 |